Numenta作為領先的腦神經科學和AI公司,正在為金融業帶來前所未有的創新和潛力。
Numenta的研究和技術旨在模擬人類大腦的工作原理,並將其應用於金融領域的數據分析和預測中,Numenta成立於2005年,由腦神經科學家Jeff Hawkins和唐·格羅賽爾(Don Grossetti)共同創辦,該公司的目標是透過研究大腦的運作方式,開發出一種稱為Hierarchical Temporal Memory(HTM)的技術,該技術模擬了大腦皮質的工作原理, HTM技術旨在處理和分析時間序列數據,這在金融領域中具有重要意義,因為金融市場的數據往往具有時間相依性和動態性。
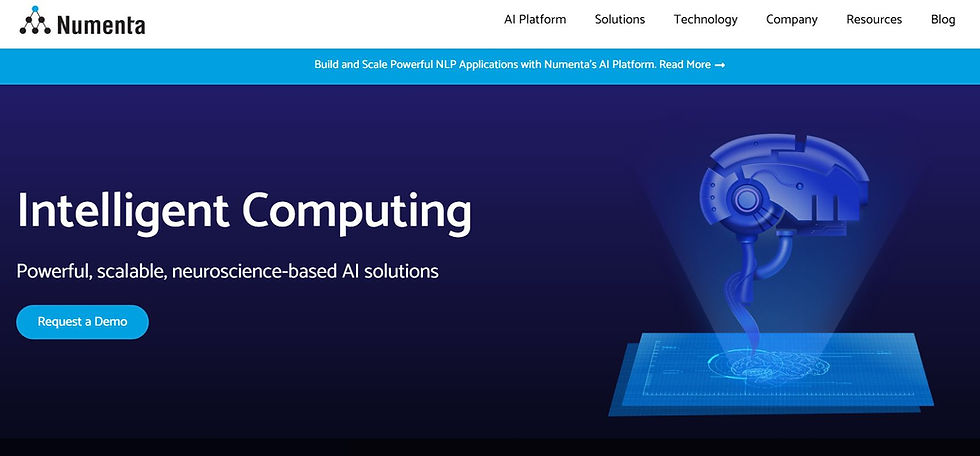
Numenta的核心技術是HTM(Hierarchical Temporal Memory,層次時間記憶)模型,該模型基於腦神經科學的原理和理論, HTM模型具有分層結構和時間記憶的特點,可以捕捉和預測時間序列數據中的模式和趨勢,該模型具有自適應學習和調整能力,可以根據新的數據和情境進行調整,提供更準確的分析和預測結果。
在競爭力的產品和服務方面,HTM模型在腦神經科學和人工智能領域具有獨特性,該模型與傳統的機器學習和統計方法有所不同,更貼近於生物學的模擬。
這使得Numenta在以下方面具有競爭力:
時間序列數據分析:HTM模型專注於處理時間序列數據,並能夠捕捉時間模式和趨勢,金融市場的預測、風險管理和交易決策等方面具有優勢。
強大的模式識別能力:HTM模型以分層結構模擬大腦皮質的組織,具有優秀的模式識別能力,能夠處理大量的數據並識別出其中的模式,提供更準確的分析結果。
自適應學習和調整:HTM模型具有自適應學習和調整的能力,可以根據新的數據和情境調整模型的連接和權重,能夠適應不斷變化的數據環境和新的情景,提供更準確和可靠的預測結果。
Numenta的核心技術HTM基於腦神經科學原理,它模擬了大腦皮質神經元之間的連接和信息處理過程,在金融數據的分析和預測方面具有獨特的優勢,在金融市場的數據分析中,HTM可以捕捉到時間序列數據中的模式和趨勢,並用於預測市場走勢和風險,HTM還能夠自適應地學習和調整模型,以應對市場變化和新的數據情況。
Hierarchical Temporal Memory(層次時間記憶),是Numenta公司開發的一種基於腦神經科學的人工智能模型。該模型旨在模擬大腦皮質的工作原理,並將其應用於數據分析和預測中。
HTM模型的核心思想是模擬大腦皮質神經元之間的連接和信息處理方式,由多個層次的神經元組成,每個層次都具有不同的功能和屬性,
HTM模型的主要特點包括:
分層結構:HTM模型以分層結構模擬大腦皮質的組織,每個層次包含一組神經元,這些神經元之間通過突觸連接,形成神經網絡。
時間記憶:HTM模型著重於處理時間序列數據,通過在神經網絡中建立對時間的敏感性和序列記憶能力,能夠捕捉到時間序列數據中的模式和趨勢。
分散表徵:HTM模型使用分散表徵來表示信息,通過在神經元之間的連接中分配權重,將信息以分散的方式存儲和處理,這使得模型能夠更高效地處理大量的數據。
自適應學習:HTM模型具有自適應學習能力,能夠根據新的數據和情境調整模型的連接和權重,模型能夠適應不斷變化的數據環境和新的情景。
HTM模型的應用在金融領域中具有潛力,如在金融市場的數據分析中,HTM模型可以捕捉到市場的短期和長期趨勢,並提供基於模式識別的交易信號和風險評估,HTM模型還可以應用於信用評級、風險管理和市場監測等金融業務領域。
需要注意的是,HTM模型是一種複雜的模型,其計算要求和運算成本相對較高,在應用和使用時,需要充分評估數據的品質、模型的參數設定和適用性,以確保模型的準確性和效能,同時,也需要考慮相關的法規和隱私問題,以確保模型的合法和合規使用。
Numenta的應用在金融領域中仍面臨一些挑戰,首先是數據的品質和可用性。金融市場的數據通常存在噪音、不完整性和不確定性,這對於HTM模型的準確性和效果提出了挑戰,HTM模型的複雜性和計算要求也是一個問題,需要強大的計算資源和適應性算法,將HTM技術應用到實際金融業務中也需要克服相關的法規和合規要求。
在交易和投資方面,HTM模型能夠捕捉到市場的短期和長期趨勢,並提供基於模式識別的交易信號和風險評估,HTM模型還可以應用於信用評級、風險管理和市場監測等金融業務領域。Numenta的HTM模型有望提供更準確、更智能的金融分析和預測工具,並需要關注相關的法規和隱私問題,以確保這些技術的合法和合規使用。
Numenta的應用吸引著以下不同類型的使用者:
金融分析師和研究人員:金融分析師和研究人員可以使用技術和工具來進行更精準的金融數據分析和預測,可以利用Numenta的HTM模型捕捉市場趨勢和模式,從而提供更準確的市場洞察和投資建議。
投資者和交易員:個人投資者和專業交易員可以利用交易決策中獲得優勢, HTM模型可以幫助他們識別市場中的交易機會、預測價格走勢,並提供更可靠的風險管理策略。
金融科技公司:金融科技公司可以使用技術來開發智能化的金融應用和工具,可以整合Numenta的HTM模型到自己的產品中,提供更智能、更準確的金融分析和預測功能。
學術界和研究機構:學術界和研究機構可以利用技術進行腦神經科學和人工智能的研究,HTM模型進行實驗和模擬,以更好地理解大腦的工作原理和人工智能的應用。
Numenta的應用通常需要一定的專業知識和技能,使用者應該在使用之前評估其功能、限制和風險,並遵守相關法規和合規要求。
Numenta專注於腦神經科學和人工智能其特色:
基於腦神經科學:腦神經科學的原理和理論,尤其是大腦皮質的運作方式,這使得Numenta的模型與傳統的機器學習和統計方法有所不同,更貼近於生物學的模擬。
分層時間記憶(HTM)模型:Numenta的核心技術是HTM模型,該模型專注於時間序列數據的處理和分析,HTM模型具有分層結構和時間記憶的特點,能夠捕捉到時間序列數據中的模式和趨勢。
自適應學習和調整:HTM模型具有自適應學習和調整的能力,可以根據新的數據和情境調整模型的連接和權重。這使得模型能夠適應不斷變化的數據環境和新的情景,並提供更準確的分析和預測。
應用於金融領域:Numenta的技術在金融領域中具有應用價值, HTM模型可以應用於金融數據分析、預測市場走勢、交易決策和風險管理等方面,其獨特的模式識別和時間序列處理能力使得金融科技領域具有差異化的競爭優勢。
開放源碼和社區合作:Numenta將其部分技術和工具開放源碼,鼓勵社區合作和共享,這促進了技術的發展和創新,同時也提供了更多交流和合作的機會。
市場應用:
金融市場預測:Numenta的HTM模型可以應用於金融市場的預測,例如一家基金管理公司使用Numenta的技術來預測股票價格的走勢,將HTM模型應用於歷史股價數據,並使用模型的預測結果來指導投資決策,這種應用可以提供更準確和及時的市場預測,幫助投資者做出更明智的投資選擇。
風險評估和監測:另一家金融機構使用Numenta的技術來進行風險評估和監測,將HTM模型應用於大量的金融交易數據,以識別潛在的風險信號和異常模式,這樣可以幫助機構更好地監測市場風險,提前做出相應的調整和應對措施,減少潛在的損失風險。
自動化交易:一些交易公司也開始探索將Numenta的技術應用於自動化交易系統中,使用HTM模型來分析市場數據,捕捉交易信號和模式,並自動執行相應的交易,這樣可以提高交易的效率和準確性,並減少人為因素對交易決策的影響。
在同質性腦神經科學和人工智能領域相關競品:
DeepMind:DeepMind是一家英國人工智能公司,專注於開發強化學習和深度學習的技術,在人工智能領域取得了重大突破,包括AlphaGo在圍棋比賽中擊敗世界冠軍。 DeepMind的技術也應用於金融領域,例如在投資組合優化和交易執行方面。
OpenAI:OpenAI是一家人工智能研究實驗室,旨在促進人工智能的發展和開放,研究領域包括自然語言處理、機器學習和強化學習等, OpenAI開發了一些具有影響力的模型,如GPT系列模型,在自然語言處理和生成方面取得了顯著成果。
IBM Watson:IBM Watson是IBM開發的一個認知計算平台,利用人工智能和自然語言處理等技術進行數據分析和智能問答,在金融領域的應用包括風險管理、客戶服務和投資決策等。
AlphaSense:AlphaSense是一個專注於金融搜索和分析的平台,利用自然語言處理和機器學習技術,幫助金融從業者更快速地搜索和分析大量的金融文檔和數據,以提供即時的市場洞察和投資建議。
這些公司和產品在不同方面與Numenta有相關性,包括人工智能、數據分析、自然語言處理等領域,請注意每個公司和產品的特點和應用領域可能有所不同,需根據具體需求進行評估和選擇。
Numenta, as a leading neuroscience and AI company, is bringing unprecedented innovation and potential to the financial industry. Numenta's research and technology aim to simulate the workings of the human brain and apply it to data analysis and prediction in the financial domain. Founded in 2005 by neuroscientists Jeff Hawkins and Don Grossetti, the company's goal is to develop a technology called Hierarchical Temporal Memory (HTM) through the study of brain operation principles. HTM technology simulates the workings of the cortical regions of the brain and is designed to handle and analyze time-series data, which is crucial in the financial field due to the time-dependency and dynamics of financial market data.
At the core of Numenta's technology is the HTM (Hierarchical Temporal Memory) model, which is based on principles and theories of neuroscience. The HTM model features a hierarchical structure and temporal memory, allowing it to capture and predict patterns and trends in time-series data. The model has adaptive learning and adjustment capabilities, enabling it to adapt to new data and contexts, providing more accurate analysis and predictions.
In terms of competitive products and services, Numenta's HTM model stands out in the fields of neuroscience and artificial intelligence. Unlike traditional machine learning and statistical methods, the HTM model closely emulates biological systems.
This gives Numenta a competitive edge in the following areas:
Time-series data analysis: The HTM model focuses on processing time-series data and can capture time patterns and trends. This advantage positions Numenta well in areas such as market forecasting, risk management, and trading decisions within the financial market.
Powerful pattern recognition capability: The HTM model simulates the hierarchical organization of the brain's cortex and exhibits excellent pattern recognition capabilities. Numenta can handle large volumes of data and identify patterns, providing more accurate analysis results.
Adaptive learning and adjustment: The HTM model has the ability to adaptively learn and adjust its connections and weights based on new data and contexts. Numenta's model can adapt to changing data environments and new scenarios, offering more accurate and reliable prediction results.
Numenta's core technology, HTM, is based on principles of neuroscience, simulating the connections and information processing within the neocortex. It possesses unique advantages in analyzing and predicting financial data. In the analysis of financial market data, HTM can capture patterns and trends in time-series data and utilize them to forecast market trends and risks. The HTM model also has the ability to adapt and adjust itself in response to market changes and new data scenarios.
Hierarchical Temporal Memory (HTM) is an artificial intelligence model developed by Numenta based on principles from neuroscience. The model aims to simulate the workings of the neocortex and applies it to data analysis and prediction.
The HTM model's key features include:
Hierarchical structure: The HTM model simulates the hierarchical organization of the neocortex, with each level consisting of a set of neurons connected through synapses to form a neural network.
Temporal memory: The HTM model focuses on processing time-series data and establishes sensitivity to time and sequential memory within the neural network. This allows it to capture patterns and trends in time-series data.
Sparse representation: The HTM model utilizes a distributed representation to store and process information. Information is stored and processed in a distributed manner through weighted connections between neurons, enabling the model to efficiently handle large amounts of data.
Adaptive learning: The HTM model has adaptive learning capabilities, allowing it to adjust its connections and weights based on new data and contexts. The model can adapt to changing data environments and new scenarios.
The HTM model has potential applications in the financial field. For example, in financial data analysis, the HTM model can capture short-term and long-term trends in the market and provide pattern-based trading signals and risk assessments. It can also be applied in credit rating, risk management, and market surveillance in the financial industry.
It is important to note that the HTM model is a complex model with relatively high computational requirements and costs. When applying and using the model, it is necessary to thoroughly evaluate data quality, parameter settings, and applicability to ensure accuracy and performance. Additionally, relevant regulations and privacy considerations need to be taken into account to ensure the lawful and compliant use of the model.
Numenta's applications in the financial domain still face some challenges. Firstly, the quality and availability of data pose challenges to the accuracy and effectiveness of the HTM model, as financial market data often contain noise, incompleteness, and uncertainty. The complexity and computational requirements of the HTM model are also considerations, requiring powerful computing resources and adaptive algorithms. Applying HTM technology to practical financial operations also requires overcoming relevant regulatory and compliance requirements.
In terms of trading and investment, Numenta's HTM model can capture short-term and long-term trends in the market and provide pattern-based trading signals and risk assessments. It can be applied in credit rating, risk management, market monitoring, and other financial business areas. Numenta's HTM model is expected to provide more accurate and intelligent financial analysis and prediction tools while considering relevant regulations and privacy issues to ensure the lawful and compliant use of these technologies.
Numenta's applications attract different types of users, including:
Financial analysts and researchers: They can utilize Numenta's technology and tools for more precise financial data analysis and prediction. By employing Numenta's HTM model to capture market trends and patterns, they can provide more accurate market insights and investment recommendations.
Investors and traders: Individual investors and professional traders can gain an advantage in their trading decisions by utilizing Numenta's applications. The HTM model can help them identify trading opportunities and predict price trends, providing more reliable risk management strategies.
Fintech companies: Fintech companies can utilize Numenta's technology to develop intelligent financial applications and tools. They can integrate Numenta's HTM model into their own products, offering more intelligent and accurate financial analysis and prediction capabilities.
Academic and research institutions: Academic and research institutions can utilize Numenta's technology for research in neuroscience and artificial intelligence. They can conduct experiments and simulations using the HTM model to better understand the workings of the brain and the applications of artificial intelligence.
The applications of Numenta typically require a certain level of expertise and skills. Users should evaluate the functionality, limitations, and risks before using them and adhere to relevant regulations and compliance requirements.
Numenta's focus on neuroscience and artificial intelligence is characterized by:
Neuroscience-based approach: Numenta's technology is based on principles and theories of neuroscience, particularly the functioning of the neocortex. This sets Numenta's models apart from traditional machine learning and statistical methods, making them more biologically inspired.
Hierarchical Temporal Memory (HTM) model: Numenta's core technology is the HTM model, which specializes in processing and analyzing time-series data. With its hierarchical structure and temporal memory, the HTM model captures patterns and trends in time-series data.
Adaptive learning and adjustment: The HTM model has the capability to adaptively learn and adjust its connections and weights based on new data. This adaptability allows the model to cope with changing data environments and new scenarios, providing more accurate analysis and predictions.
Application in the financial field: Numenta's technology has valuable applications in the financial domain. The HTM model can be applied to financial data analysis, market trend forecasting, trading decisions, and risk management. Its unique pattern recognition and time-series processing capabilities give Numenta a differentiated competitive advantage in the fintech sector.
Open-source and community collaboration: Numenta open-sources a portion of its technology and tools, encouraging community collaboration and sharing. This promotes the development and innovation of the technology while providing more opportunities for communication and cooperation.
Market applications:
Financial market forecasting: Numenta's HTM model can be applied to market forecasting, such as a fund management company using Numenta's technology to predict stock price trends. By applying the HTM model to historical stock price data and utilizing its prediction results, more accurate and timely market forecasts can be provided to assist investors in making wiser investment choices.
Risk assessment and monitoring: Another financial institution utilizes Numenta's technology for risk assessment and monitoring. By applying the HTM model to a large volume of financial transaction data, potential risk signals and abnormal patterns can be identified. This helps the institution better monitor market risks, make proactive adjustments, and mitigate potential losses.
Automated trading: Some trading firms are exploring the application of Numenta's technology in automated trading systems. By using the HTM model to analyze market data, capture trading signals and patterns, and automatically execute corresponding trades, trading efficiency and accuracy can be improved while reducing the impact of human factors on trading decisions.
In the field of homogenous neuroscience and artificial intelligence, there are related competitors such as:
DeepMind: DeepMind, a British AI company, focuses on developing technologies in reinforcement learning and deep learning. It has achieved significant breakthroughs in the field of artificial intelligence, including AlphaGo defeating the world champion in the game of Go. DeepMind's technology is also applied in the financial domain, such as portfolio optimization and trade execution.
OpenAI: OpenAI is an AI research laboratory that aims to promote the development and openness of artificial intelligence. Research areas include natural language processing, machine learning, and reinforcement learning. OpenAI has developed influential models like the GPT series, achieving significant results in natural language processing and generation.
IBM Watson: IBM Watson is a cognitive computing platform that utilizes AI and natural language processing for data analysis and intelligent question answering. Its applications in the financial domain include risk management, customer service, and investment decisions.
AlphaSense: AlphaSense is a platform focused on financial search and analysis. It leverages natural language processing and machine learning techniques to help financial professionals quickly search and analyze a large amount of financial documents and data, providing real-time market insights and investment recommendations.
These companies and products have relevance to Numenta in various aspects, including artificial intelligence, data analysis, and natural language processing. It is important to note that each company and product may have different characteristics and application areas. Evaluation and selection should be based on specific needs.
#Numenta #腦神經科學 #人工智能 #HTM模型 #分層時間記憶 #時間序列數據分析 #模式識別 #自適應學習 #預測 #金融科技 #neuroscience #artificialintelligence #HTMmodel #hierarchicaltemporalmemory #timeseriesdataanalysis #patternrecognition #adaptivelearning #prediction #fintech