「智能製造」新時代:運用人工智慧打造製造生產排程與精益管理
- Arthur Martinez
- 2023年11月22日
- 讀畢需時 5 分鐘
已更新:2023年11月24日
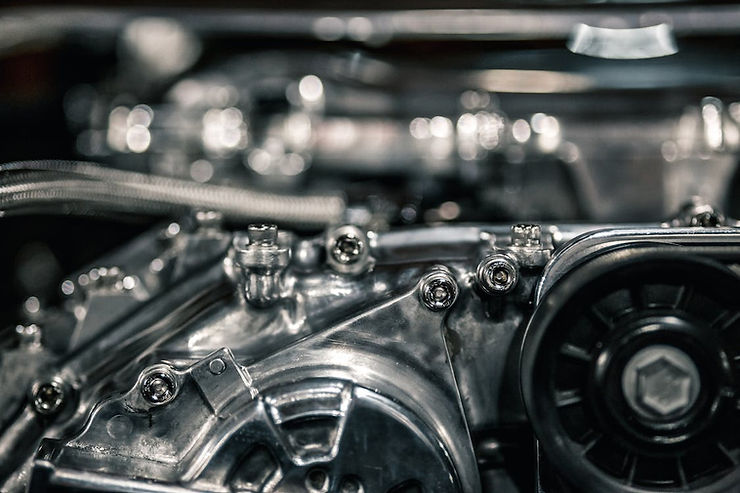
隨著人工智慧技術的不斷發展,越來越多的企業開始探索如何將其應用於生產管理領域,以實現更高效率、更低成本的製造流程。其中,製造生產排程與精實管理是應用人工智慧技術的兩大重要領域。本文將探討如何運用人工智慧技術,實現製造生產排程與精實管理的最佳實踐。
首先,讓我們來瞭解一下人工智慧在製造生產排程中的應用。傳統的生產排程往往依賴於經驗和直覺,並且在處理複雜的製造過程時存在著困難。然而,人工智慧技術的出現為生產排程帶來了全新的思路。通過利用機器學習演算法,我們可以從大量的歷史數據中學習,並將這些經驗應用於新的生產排程中。這種方法可以有效地提高生產排程的準確性和效率,並減少生產停機時間和延誤交貨等問題。
在實踐中,我們可以使用各種開源的機器學習框架和庫,例如Python的Scikit-learn和TensorFlow等,以實現生產排程的自動化。通過適當的特徵選擇和模型訓練,我們可以開發出高效的生產排程模型,並實現對生產過程的智能監控和預測,從而實現更優秀的生產計劃。
除了生產排程外,精益生產管理也是應用人工智慧技術的一個重要領域。精益生產管理是一種強調減少浪費、提高效率和降低成本的生產管理方法。在實踐中,我們可以利用人工智慧技術,實現精益生產管理的最佳實踐。精益生產管理是一種以提高價值流動效率為目標的生產管理方式。其核心思想是消除浪費,包括等待、庫存、瑕疵品、不必要的運動、過度生產、運輸和不必要的過程。通過這種方法,可以更好地滿足客戶需求,提高產品質量和交貨速度,同時降低成本和風險。
精益生產管理源自於日本豐田汽車公司的生產方式,也稱為豐田生產系統。它強調生產過程的平衡、流暢性和持續改進。在精益生產管理中,每個生產過程都被認為是一個價值流程,並且要經過一系列的分析、優化和持續改進才能實現更高的價值流動效率。
精益生產管理方法包括: 1.價值流分析(Value Stream Mapping):對生產過程進行全面評估,分析價值流動情況,找出浪費點。
2.流程優化(Flow Optimization):通過優化流程和減少浪費,提高生產效率和產品質量。
3.持續改進(Continuous Improvement):不斷測試和改進生產過程,以實現更高的效率和更低的成本。
4.拉動生產(Pull Production):生產基於需求的產品,而不是基於預測,通過監控庫存水準以及適時補貨,以實現高效的生產。
5.人員培訓(Employee Training):精益生產管理需要團隊成員具備組織改善和問題解決的技能,以支持持續改進和高效率的生產。
精益生產管理已被廣泛應用於各種行業,包括汽車、電子、製造和醫療保健等。它是一種既簡單又有效的方法,可以幫助企業提高效率和產品質量,同時降低成本和風險。 除了生產排程方面,AI也可以應用於精實管理的各個方面,例如品質管理、設備維護和物流管理等。舉例來說,在品質管理方面,AI可以分析大量的產品檢測數據,以預測可能出現的產品缺陷,從而提前進行調整和改進,減少廢品率和產品召回率。在設備維護方面,AI可以應用於設備故障預測和維修排程,以提高設備的使用效率和延長設備的壽命。在物流管理方面,AI可以分析交通和天氣等數據,以優化物流路線和配送計劃,減少時間和成本。
人工智慧在製造生產排程和精益管理方面有著廣泛的應用前景。AI可以幫助企業實現生產效率和質量的提升,同時也可以降低成本和風險。AI技術的應用,可以幫助企業在激烈的市場競爭中取得優勢,實現可持續發展。
Scikit-learn和TensorFlow是目前廣泛使用的機器學習庫和框架。它們可以幫助工廠管理者和AI工程師實現對生產過程的智慧監控和預測。以下是一些實際的應用範例:
1. Scikit-learn應用於資料探勘和監控
Scikit-learn可以應用於生產過程中的資料探勘和監控。例如,一家汽車製造商使用Scikit-learn來監控每個製造過程的質量,從而提高整個生產線的效率。他們使用Scikit-learn建立了一個預測模型,將生產過程中的資料傳輸到該模型,以確定產品是否符合質量標準。該模型還可以通過分析數據來發現任何產品製造過程中的異常情況,並通過調整生產線來解決問題。
2. TensorFlow應用於智能監控和預測
TensorFlow可以應用於生產過程中的智慧監控和預測。例如,一家化工公司使用TensorFlow來監控其生產過程中的溫度、壓力和其他參數。他們使用TensorFlow建立了一個深度學習模型,將生產過程中的數據傳輸到該模型,以進行預測和監控。通過該模型,他們可以發現任何潛在的生產問題,並在生產線上及時解決。
3. Scikit-learn和TensorFlow結合應用
Scikit-learn和TensorFlow也可以結合應用於生產過程中的智能監控和預測。例如,一家電子產品製造商使用Scikit-learn和TensorFlow建立了一個結合的監控系統,用於監控其生產過程中的每個步驟,包括物料進貨、製造過程、檢驗和包裝。該系統使用Scikit-learn進行資料探勘和監控,以確定生產過程中的問題,然後使用TensorFlow進行預測,以預測任何潛在的問題。
Smart Manufacturing" New Era: Utilizing Artificial Intelligence to Build Manufacturing Production Scheduling and Lean Management
As artificial intelligence technology continues to advance, more and more companies are exploring how to apply it to the field of production management, aiming to achieve more efficient and cost-effective manufacturing processes. Among various applications, two crucial areas for applying artificial intelligence technology are manufacturing production scheduling and lean management. This article will explore the best practices in utilizing artificial intelligence technology for achieving optimal manufacturing production scheduling and lean management.
Firstly, let's delve into the application of artificial intelligence in manufacturing production scheduling. Traditional production scheduling often relies on experience and intuition, facing difficulties in handling complex manufacturing processes. However, the emergence of artificial intelligence technology brings a new perspective to production scheduling. By leveraging machine learning algorithms, we can learn from extensive historical data and apply this experience to new production schedules. This approach can effectively enhance the accuracy and efficiency of production scheduling, reducing issues such as production downtime and delayed deliveries.
In practice, various open-source machine learning frameworks and libraries, such as Scikit-learn and TensorFlow in Python, can be utilized to automate production scheduling. Through proper feature selection and model training, we can develop efficient production scheduling models, enabling intelligent monitoring and prediction of the production process for superior production planning.
In addition to production scheduling, lean production management is another crucial area for applying artificial intelligence technology. Lean production management is a method that emphasizes reducing waste, improving efficiency, and lowering costs. In practice, we can use artificial intelligence technology to implement best practices in lean production management. Originating from the Toyota Production System in Japan, lean production management focuses on balancing, smoothness, and continuous improvement of the production process. In this method, each production process is considered a value stream, undergoing a series of analyses, optimizations, and continuous improvements to achieve higher value stream efficiency.
Lean production management methods include:
Value Stream Mapping: Comprehensive evaluation of production processes, analyzing the flow of value and identifying waste points.
Flow Optimization: Improving production efficiency and product quality by optimizing processes and reducing waste.
Continuous Improvement: Constant testing and improvement of production processes to achieve higher efficiency and lower costs.
Pull Production: Producing products based on demand rather than predictions, efficiently monitoring inventory levels, and replenishing timely to achieve effective production.
Employee Training: Lean production management requires team members to possess skills in organizational improvement and problem-solving to support continuous improvement and high efficiency in production.
Lean production management has been widely applied across various industries, including automotive, electronics, manufacturing, and healthcare. It is a simple yet effective method that helps businesses enhance efficiency and product quality while reducing costs and risks.
Apart from production scheduling, AI can also be applied to various aspects of lean management, such as quality management, equipment maintenance, and logistics management. For instance, in quality management, AI can analyze extensive product inspection data to predict potential product defects, enabling adjustments and improvements in advance to reduce defect rates and product recalls. In equipment maintenance, AI can be applied to equipment failure prediction and maintenance scheduling, improving equipment efficiency and extending equipment lifespan. In logistics management, AI can analyze data such as traffic and weather to optimize logistics routes and delivery plans, reducing time and costs.
The application prospects of artificial intelligence in manufacturing production scheduling and lean management are extensive. AI can assist businesses in achieving improvements in production efficiency and quality while lowering costs and risks. The application of AI technology can help businesses gain a competitive advantage in the fierce market competition, facilitating sustainable development.
Scikit-learn and TensorFlow are widely used machine learning libraries and frameworks. They can assist factory managers and AI engineers in achieving intelligent monitoring and prediction of the production process. Here are some practical application examples:
Scikit-learn Application in Data Mining and Monitoring Scikit-learn can be applied to data mining and monitoring in the production process. For example, an automotive manufacturer uses Scikit-learn to monitor the quality of each manufacturing process, thereby improving the efficiency of the entire production line. They use Scikit-learn to build a predictive model, transmitting production process data to the model to determine if the product meets quality standards. The model can also identify any abnormalities in the production process by analyzing data, enabling adjustments to be made to the production line promptly.
TensorFlow Application in Intelligent Monitoring and Prediction TensorFlow can be applied to intelligent monitoring and prediction in the production process. For instance, a chemical company uses TensorFlow to monitor parameters such as temperature and pressure in its production process. They use TensorFlow to build a deep learning model, transmitting process data to the model for prediction and monitoring. Through this model, they can identify potential production issues and address them in real-time on the production line.
Combined Application of Scikit-learn and TensorFlow Scikit-learn and TensorFlow can also be combined for intelligent monitoring and prediction in the production process. For example, an electronics manufacturer combines Scikit-learn and TensorFlow to establish a integrated monitoring system for every step of its production process, including material procurement, manufacturing, inspection, and packaging. The system uses Scikit-learn for data mining and monitoring to identify issues in the production process, and then utilizes TensorFlow for prediction to foresee potential problems.
#payrise #sidejob #sidehustlejobs #sidejobs #sidehustle #hustle #DoorDash #remoteworkers #遠距工作 #more_vert #remote jobs #生產管理 #製造生產排程 #精益管理 #機器學習演算法 #生產排程準確性 #生產停機時間 #延誤交貨 #開源機器學習框架 #Scikit-learn #TensorFlow #自動化生產排程 #特徵選擇 #模型訓練 #智能監控 #預測模型 #精益生產管理 #價值流動效率 #消除浪費 #持續改進 #拉動生產 #人員培訓 #生產效率 #產品質量 #成本降低 #風險降低 #品質管理 #設備維護 #物流管理 #市場競爭 #可持續發展 #工廠管理 #AI工程師 #資料探勘 #智慧監控 #預測分析 #深度學習模型 #結合應用 #生產過程 #artificialintelligence #productionmanagement #manufacturingprocess #productionscheduling #leanmanagement #machinelearningalgorithms #open-sourceframeworks #Scikit-learn #TensorFlow #automatedproductionscheduling #featureselection #modeltraining #intelligentmonitoring #prediction #valuestreamanalysis #flowoptimization #continuousimprovement #pullproduction #employeetraining #ToyotaProductionSystem #wasteelimination #efficiencyimprovement #costreduction #qualitymanagement #equipmentmaintenance #logisticsmanagement #productinspection #defectprediction #equipmentfailureprediction #maintenancescheduling #logisticsroutesoptimization #competitiveadvantage #sustainabledevelopment #Scikit-learnapplication #datamining #monitoring #TensorFlowapplication #intelligentmonitoring #combinedapplication #integration #stepbystepmonitoring #potentialproblemsprediction